In today's dynamic legal landscape, the demands on law firms are evolving rapidly. Introducing Canon Legal 2030, a visionary program by Canon Business Process Services, designed to redefine and enhance the operational efficiency of your law firm. Canon Legal 2030 addresses the critical needs of modern law firms, ensuring they are equipped to thrive in a hybrid work environment. You can elevate your firm's operations with Canon Legal 2030.
Seamless Integration and Support
With Canon Legal 2030, enjoy the peace of mind that comes with having a dedicated on-site team available five days a week. We ensure seamless integration of our services, allowing your attorneys to work efficiently, whether remotely or in the office.
Tailored Solutions for Unique Challenges
Every law firm is unique, and so are its operational challenges. Canon Legal 2030 provides customized solutions that address specific needs, from resource management to service delivery. By partnering with us, you can alleviate the pressures of in-house management and focus on driving growth and innovation.
Achieve More with Outsourced Office Support
Transitioning to an outsourced office support model can bring significant business benefits. Canon Legal 2030 offers the flexibility and innovation needed to stay ahead in the competitive legal industry. By outsourcing these functions, your firm can reduce operational complexities and focus on core competencies.
Discover how Canon Legal 2030 can transform your firm's operations and elevate your service delivery. Our vision is to empower law firms to achieve their strategic objectives through optimized resources and enhanced workplace experiences.
Client Testimonial: Law Firm Wins With Canon Digital Mailroom
A leading law firm struggling to handle thousands of pieces of mail daily, partnered with Canon to digitally transform their mailroom. With an improved digital mailroom, they eliminated nearly all overtime saving time and money for the firm. With the documents being scanned and sent to users in near-time, they were able to work smarter, faster, and more securely.
Canon Innovates with New Support Models for the Hybrid Office
To optimize operations and innovate, law firms are increasingly seeking outside assistance. We can help your firm quickly evolve its workplace and support service delivery to meet the challenges that are rapidly changing how law firms work. This evolution requires a shift from traditional to transformative office, facility and support services. The right partner can help you successfully manage this shift. This includes creating and leveraging best practices, new workplace support models, event planning, health and wellness program planning, and digital enablement and transformation implementations. As you consider this partnership, it is important to know what you should look for in a managed services provider.
A Customized, On-site Approach to Optimizing Your Firm’s Essential Work Culture
One important difference in our approach is that Canon has the flexibility to deliver customized law firm support services, on-site at your location. In addition to implementing a leading-edge, tailored solution that supports your specific hybrid office needs we offer an elevated approach to legal managed services. This advanced approach includes an elevated and integrated approach to reception, hospitality, facility and office services, document services, mail/messenger, procurement/vendor management, enterprise print and device management, records management, and legal to business administrative services.
Canon Elevates Workplace Experience For Am Law-ranked Law Firm
Canon helped a prominent, Am Law-ranked law firm successfully move into their new offices while implementing an unparalleled, innovative workplace experience. Canon transformed their onsite program by deploying new technologies and best practices as well as by elevating the firm's office services, including mail, print and facilities management. With the goal of maintaining services and staff, Canon retained the existing workforce while implementing skills development and mentoring, cross-training and new career growth opportunities for the team.
Law Firm Support and Transformation Services Can Give You A Competitive Advantage
Canon Supports Your Initiatives Enabling Your Knowledge Workers To Work from Anywhere
From space management, to workplace experience, to administration and business services, Canon provides the support you need to advance your workplace experience.
Canon provides an extension of the on-site teams to virtual services for technology support, concierge and reception services to deliver a convenient, intuitive overall experience.
Canon’s Floor Ambassador Program Delivers on Law Firm’s Mission
An Am Law-ranked law firm tapped Canon to provide a personalized, consistent, white-glove service throughout their U.S. offices. With a workplace services ambassador program, Canon strives to anticipate the firm’s needs and exceed expectations. This is a prime example of how Canon elevates law office support services to a new level.
Innovative Law Office Support Services Advance the Workplace Experience
A leading international law firm launched an initiative to meet two key challenges. One was connected with the planned relocation of the firm’s corporate headquarters to a new site. The goal was to engage a managed services provider to help the firm reduce a significant amount of paper documents accumulated over decades of being in the same location; paper that the firm didn’t want to bring to the new site. The firm’s other challenge, also related to the move, was to consolidate its office services program from two managed services providers to one. The firm wanted one partner that had the expertise to oversee its mail, print/copy, office services and records management operations as well as enhance the end user experience through a customized services program.
A strategy was developed for the on-site delivery of critical services. Canon showed that it could deliver on this initiative based on experienced on-site and off-site personnel and a detailed project plan. As a result, the firm engaged Canon for document scanning. While the firm was considering proposals for office services, Canon was building credibility based on how its on-site team was managing the office move/scanning project. Canon’s team worked closely and efficiently with the firm’s administrative personnel and business stakeholder; effectively managed logistics; successfully coordinated Canon’s off-site support capabilities; and consistently maintained smooth, streamlined workflows. This proven credibility, plus the ability to meet the firm’s objectives, contributed to the firm awarding Canon the office services contract for all of the firm’s locations.
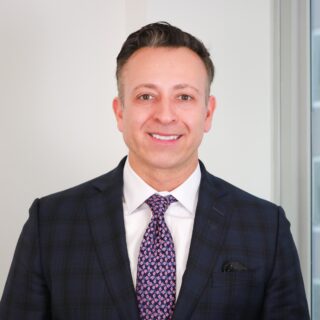
We Help Legal Organizations Leverage Transformative Approaches and Technology Solutions
"Our clients count on us to help identify areas within the firm that better prepare them for the future of work. With more than 30 years of experience serving the legal community, we are the go-to provider for on-site workplace experience services, administrative and business support services, print and mail center services. Our teams are dedicated to providing the highest level of service and truly value the client relationships we have built over the years."
Scott Paster
Vice President